The Comprehensive Beginner’s Guide to Analyzing Data for Success
Introduction to Data Analysis
Data analysis is an integral component of modern business strategy. In a digital age where information is abundant, understanding how to effectively analyze data can be the difference between success and failure for businesses. Whether you’re a seasoned professional or just dipping your toes into the world of data analysis, this comprehensive guide will equip you with the knowledge and tools necessary to navigate this complex field.
Understanding the Basics
What is Data Analysis?
Data analysis is the process of examining, cleaning, transforming, and modeling data to discover useful information, draw conclusions, and support decision-making. It involves a variety of techniques and methods to uncover patterns, trends, and insights hidden within datasets.
Why is Data Analysis Important?
Data analysis plays a crucial role in various aspects of business operations, including marketing, sales, finance, operations, and more. By leveraging data-driven insights, organizations can make informed decisions, identify opportunities for growth, mitigate risks, optimize processes, and gain a competitive edge in the market.
Getting Started with Data Analysis
Setting Objectives
Before diving into data analysis, it’s essential to define clear objectives and goals. What are you trying to achieve through data analysis? Whether it’s improving customer retention, optimizing marketing campaigns, or enhancing product offerings, establishing specific objectives will guide your analysis and ensure actionable outcomes.
Data Collection and Preparation
The first step in data analysis is gathering relevant data from various sources. This may include internal databases, external APIs, surveys, social media platforms, and more. Once collected, the data must be cleaned and preprocessed to remove inconsistencies, errors, and duplicates, ensuring accuracy and reliability.
Exploratory Data Analysis (EDA)
Exploratory Data Analysis (EDA) is a critical phase where analysts explore and visualize the data to gain initial insights and identify patterns. Techniques such as summary statistics, data visualization, and correlation analysis help uncover relationships between variables and highlight key trends within the dataset.
Statistical Analysis
Statistical analysis involves applying mathematical models and techniques to quantify relationships and make predictions based on the data. This may include hypothesis testing, regression analysis, time series analysis, clustering, and classification algorithms, depending on the nature of the data and the objectives of the analysis.
Advanced Data Analysis Techniques
Predictive Modeling
Predictive modeling involves using historical data to forecast future outcomes or trends. By building predictive models using machine learning algorithms such as linear regression, decision trees, or neural networks, organizations can anticipate customer behavior, identify potential risks, and make proactive decisions to drive business growth.
Sentiment Analysis
Sentiment analysis is a technique used to analyze text data and determine the sentiment or opinion expressed within it. By leveraging natural language processing (NLP) algorithms, organizations can gain insights into customer feedback, social media conversations, and online reviews to understand customer sentiment and tailor their strategies accordingly.
Time Series Analysis
Time series analysis is a method used to analyze temporal data and uncover patterns or trends over time. This technique is particularly useful for forecasting future values based on historical data, identifying seasonality, trends, and anomalies, and making data-driven decisions in areas such as sales forecasting, stock market analysis, and demand planning.
Conclusion
In conclusion, data analysis is a powerful tool that enables organizations to unlock valuable insights, drive strategic decision-making, and achieve business success in today’s data-driven world. By understanding the basics of data analysis, mastering advanced techniques, and leveraging the right tools and technologies, businesses can gain a competitive edge and thrive in an increasingly complex and competitive landscape.
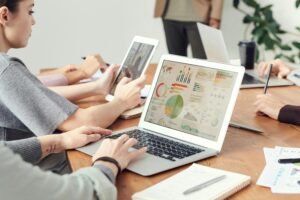
Advanced Data Analysis Tools and Technologies
Data Visualization Tools
Data visualization tools such as Tableau, Power BI, and Google Data Studio are essential for creating interactive and insightful visualizations that facilitate data exploration and communication. These tools enable analysts to present complex data in a visually appealing manner, making it easier for stakeholders to understand and interpret the findings.
Big Data Analytics
With the proliferation of big data, organizations are increasingly turning to advanced analytics techniques to extract value from large and complex datasets. Technologies such as Apache Hadoop, Apache Spark, and cloud-based platforms like Amazon Web Services (AWS) and Google Cloud Platform (GCP) provide scalable and cost-effective solutions for processing, analyzing, and deriving insights from massive volumes of data.
Machine Learning and AI
Machine learning and artificial intelligence (AI) are revolutionizing the field of data analysis by automating repetitive tasks, uncovering hidden patterns, and making predictions based on data. From recommendation systems and predictive maintenance to fraud detection and image recognition, machine learning algorithms such as random forests, support vector machines, and deep learning neural networks are powering innovative solutions across industries.
Data Mining
Data mining is a technique used to discover patterns and relationships within large datasets by applying algorithms and statistical methods. It involves tasks such as association rule mining, clustering, and classification, which help uncover valuable insights and actionable patterns hidden within the data. Data mining techniques are widely used in areas such as customer segmentation, market basket analysis, and churn prediction.
Best Practices for Effective Data Analysis
Define Clear Objectives
Before embarking on a data analysis project, it’s crucial to define clear objectives and goals. Clearly articulating what you hope to achieve through the analysis will guide your approach and ensure that the insights generated are relevant and actionable.
Use a Structured Approach
A structured approach to data analysis involves breaking down the problem into manageable steps, from data collection and preprocessing to exploratory analysis and modeling. Following a systematic process ensures that no important steps are overlooked and that the analysis is conducted in a rigorous and methodical manner.
Validate Findings
Validation is an essential step in the data analysis process to ensure the accuracy and reliability of the findings. This may involve cross-referencing results with external sources, conducting sensitivity analyses, or performing hypothesis tests to confirm the robustness of the conclusions.
Communicate Results Effectively
Effective communication of results is critical to ensuring that the insights generated from data analysis are understood and acted upon by stakeholders. Using clear and concise language, visualizations, and storytelling techniques can help convey complex findings in a compelling and accessible manner.
Conclusion
In conclusion, mastering the art of data analysis is essential for organizations looking to thrive in today’s data-driven world. By leveraging advanced techniques, tools, and technologies, businesses can unlock valuable insights, drive strategic decision-making, and gain a competitive edge in their respective industries. Whether you’re a beginner or an experienced analyst, continuously refining your skills and staying abreast of the latest developments in the field is key to success in data analysis.